Our latest deep-learning model, RFdiffusion2, can be used to generate enzyme backbones with custom active sites from simple descriptions of chemical reactions. This removes long-standing barriers to creating catalysts for plastic degradation and more.
“There’s been a lot of excitement around AI in science, but what’s happening in protein design is truly unprecedented,” said Jason Yim, a graduate student at MIT who co-led the project. “What excites me most is that we’re not just predicting structures anymore—we’re building functional molecules from first principles.”
Atom level enzyme active site scaffolding using RFdiffusion2
Published in: bioRxiv
Authors: Woody Ahern, Jason Yim, Doug Tischer, Saman Salike, Seth Woodbury, Donghyo Kim, Indrek Kalvet, Yakov Kipnis, Brian Coventry, Han Altae-Tran, Magnus Bauer, Regina Barzilay, Tommi Jaakkola, Rohith Krishna, David Baker
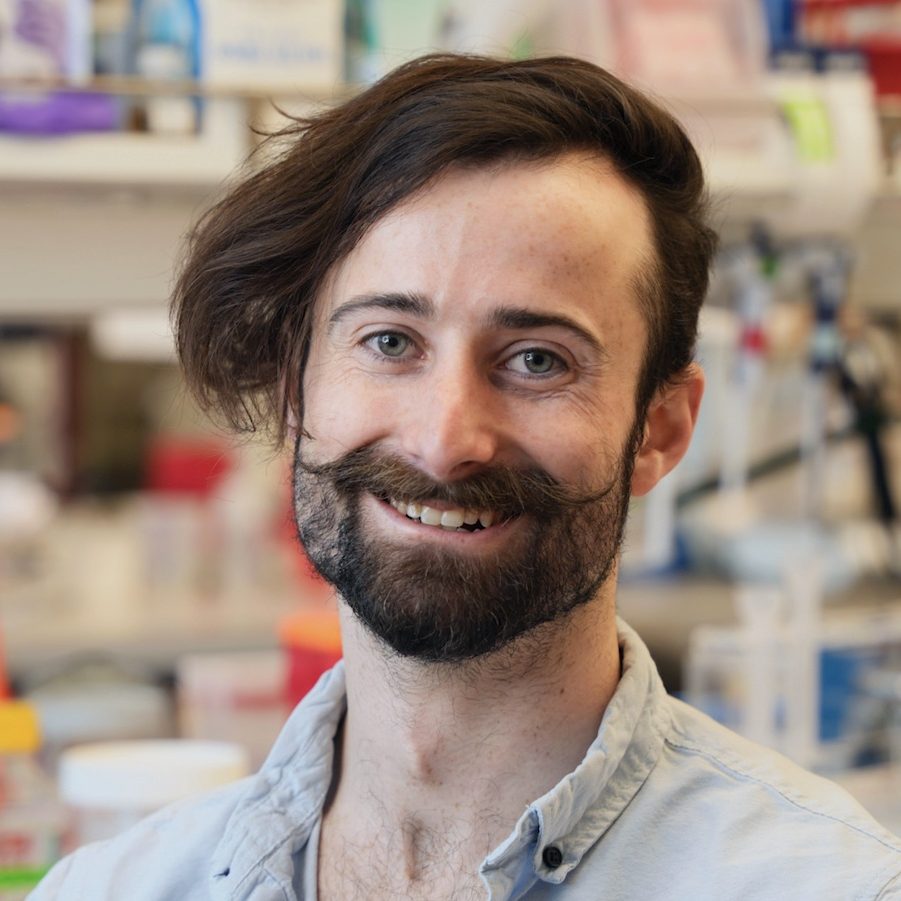
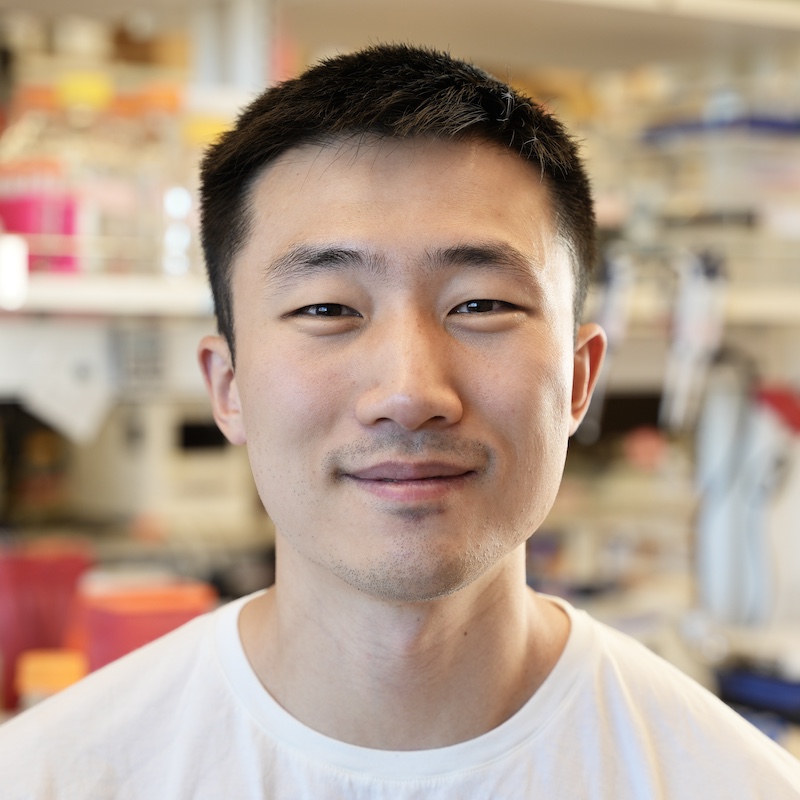
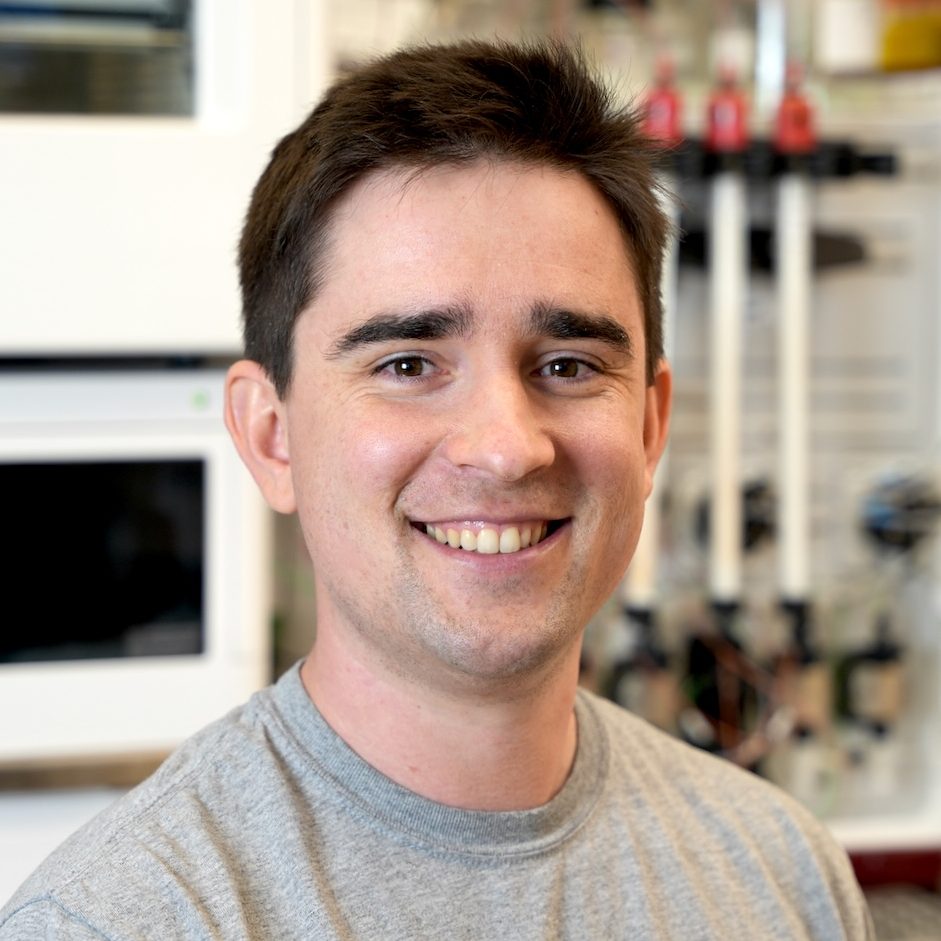
What is enzyme design?
Enzymes are the tiny protein catalysts that power all life and many industries. By speeding up chemical reactions, they help digest food, clean wastewater, manufacture medicines, and more. Developing new enzymes is challenging because these molecules must do something incredibly precise: position key atoms within an active site in precise ways to allow reactions to happen faster. Until now, that level of design precision has been extremely challenging.
Enter RFdiffusion2
Building on previous AI models from the Baker Lab and DiMaio Lab at the IPD and the Barzilay Lab and Jaakkola Lab at MIT, RFdiffusion2 uses deep learning to create protein structures tuned to catalyze specific chemical reactions. It works from a simple input—a desired chemical transformation—and generates complete backbones with active sites that can carry it out.
pre-specifying sequence indices or enumerating side chain rotamers.
Unlike previous methods, it designs enzymes without the need for experts to hand-pick a full set of atomic details for the active site. RFdiffusion2 can scaffold minimally defined catalytic sites—known as theozymes—into brand new protein structures without requiring indexed atomic positions or preset rotamers, enabling greater flexibility and diversity in design. This flexibility was achieved through machine learning, a branch of artificial intelligence.
RFdiffusion2 also introduces technical improvements like flow matching training and the ability to infer rotamers and residue indices, which allow the model to handle unindexed atomic motifs. These innovations enable a broader range of active site geometries and unlock new applications in enzyme design.
“RFdiffusion2 offers a new approach to building enzymes guided by reaction chemistry rather than existing protein structures,” said Woody Ahern, a graduate student in the Baker Lab who co-led the project. “This is what a lot of the previous work in AI-driven protein design has been building to.”
Model Performance
In silico, RFdiffusion2 outperforms previous tools. It solved every case in a new benchmark of 41 challenging enzyme design problems, evaluated using the Atomic Motif Enzyme (AME) benchmark based on the M-CSA database. The previous best tool solved only 16.
In lab tests, RFdiffusion2 successfully produced active enzymes for five distinct chemical reactions. Fewer than 100 designs were tested per case, a stark departure from traditional workflows that often require screening many thousands of molecules before finding one that performs as intended.
The team ran successful campaigns for multiple catalytic sites, including retroaldolase, cysteine hydrolase, and zinc hydrolase. The zinc hydrolases designed using RFdiffusion2 exhibited orders-of-magnitude higher activity than previously engineered metallohydrolases.
“RFdiffusion2 has allowed us to create enzymes in weeks that begin to rival those that evolved over billions of years,” said Seth Woodbury, a Baker Lab graduate student and co-lead author of a recent metallohydrolase design preprint.
Why This Matters
RFdiffusion2 is another step toward custom enzymes that degrade plastics, manufacture drugs, or carry out any number of complex reactions. The team will be making the model, training data, and benchmark set freely available to the research community.