Researchers at the Institute for Protein Design have developed a new AI-driven molecular design tool that generates completely novel bioactive peptides. The ability to create such molecules by computation alone may accelerate drug development.
RFpeptides leverages deep learning to design ring-shaped peptides called macrocycles that bind to disease-associated proteins using only the structure or sequence of a target. This is a departure from traditional peptide discovery methods, which often require extensive screening of vast peptide libraries to identify potential binders.
Introduced as a preprint, RFpeptides was developed in the laboratories of three IPD faculty members: Gaurav Bhardwaj, Frank DiMaio, and 2024 Nobel laureate David Baker. Trainees Stephen Rettie, David Juergens, and Victor Adebomi led this project.
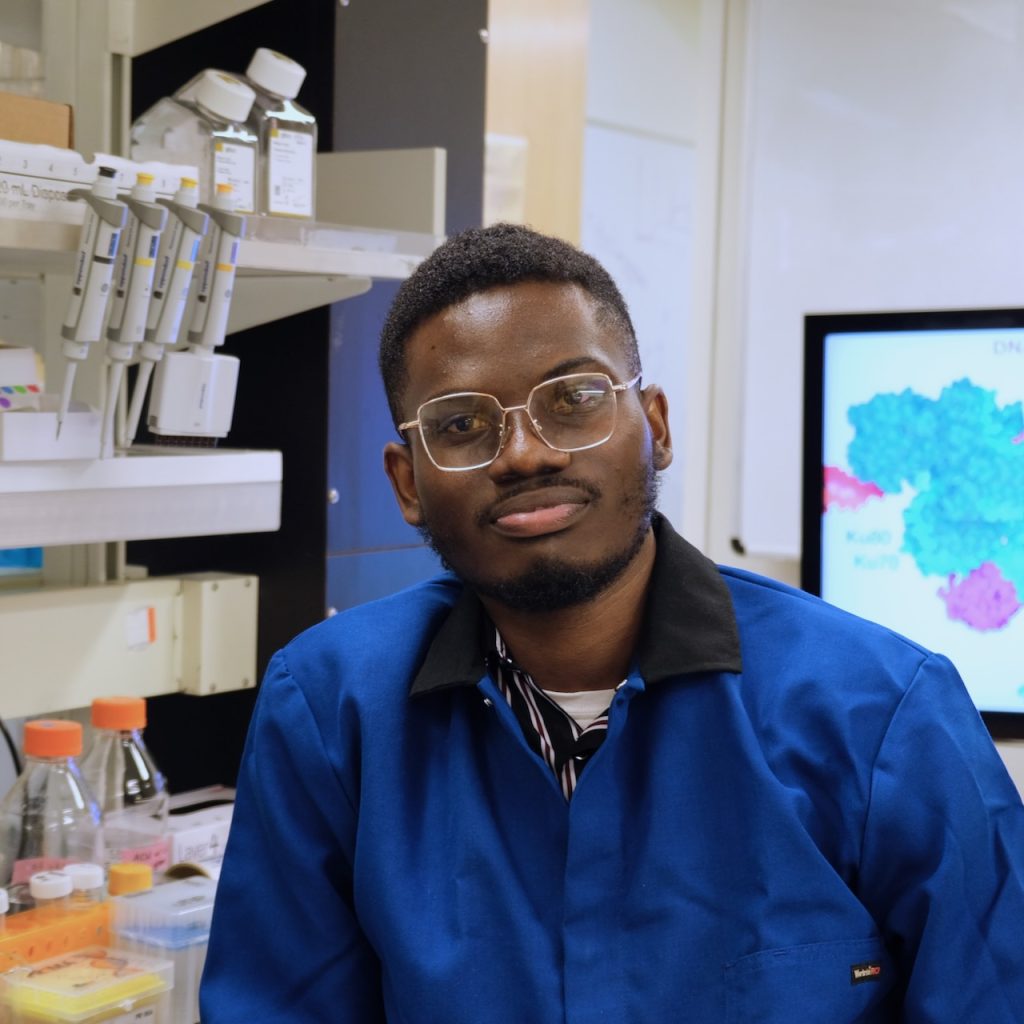
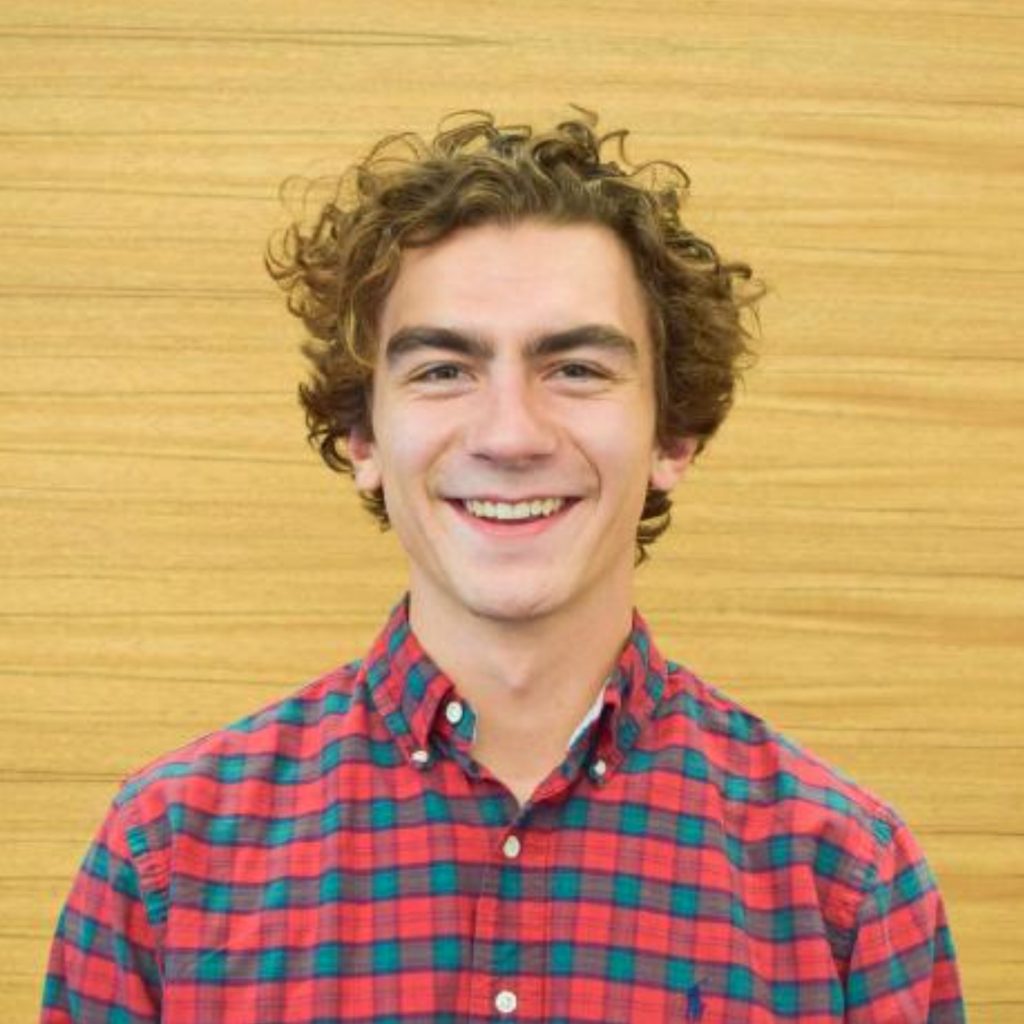
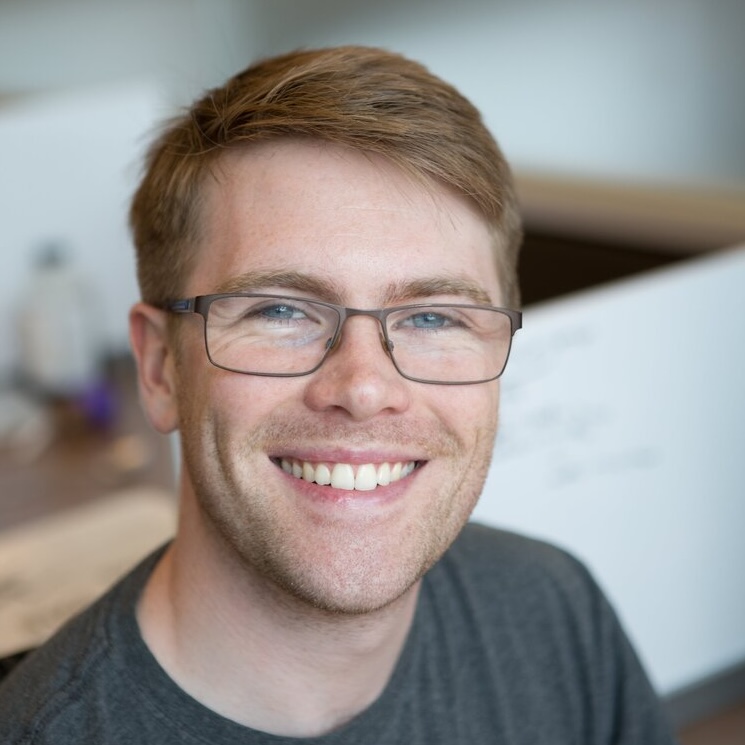
“RFpeptides extends the AI revolution in biology to the important challenge of peptide design. We hope it will help researchers create peptide-based medicines for a variety of diseases that have no good treatment options today,” said Gaurav Bhardwaj, an assistant professor of medicinal chemistry at the UW School of Pharmacy.
The University of Washington has licensed RFpeptides to Vilya, an IPD spinout company. Bhardwaj and Baker are co-founders, advisors, and shareholders of the company.
What is RFpeptides?
RFpeptides is a software tool for designing bioactive peptides with precise 3D structures. Peptides are molecules composed of only a few amino acid building blocks. When the first and last of these building blocks link together, the peptide forms a cyclic structure. This often makes the peptide more resistant to degradation and can confer other biochemical benefits, such as a more rigid structure for higher affinity target binding. The precise nature of these binding interactions can activate or deactivate a target protein and thus drive a biological effect.
How does it work?
RFpeptides builds upon the success of RFdiffusion and introduces key innovations tailored for the specific challenges of macrocyclic peptide design. Pioneered at the IPD, both tools draw inspiration from popular AI image generators that use diffusion models to synthesize new images based on user prompts. With RFdiffusion, a diffusion model sculpts clouds of disconnected amino acids into plausible biochemical structures. To create RFpeptides, the team modified the open-source tool to ensure the first and last amino acids in a designed molecule could form a chemical bond.
“By expanding the structure modeling capabilities of RoseTTAFold2 and harnessing ProteinMPNN for sequence design, we’ve created a peptide design pipeline that’s both computationally efficient and incredibly accurate,” said associate professor of biochemistry Frank DiMaio.
Testing RFpeptides
To demonstrate that RFpeptides can produce functional binding peptides for a range of challenging targets, the team selected four proteins implicated in hospital-derived bacterial infection, cancer, and other cellular processes important to human health. They synthesized and tested over a dozen designed macrocycle binders, identifying high-affinity interactions with each target.
“The highlight for me was that we successfully produced a high-affinity binder for a target with no known structure. Starting from just the target’s amino acid sequence, we predicted its structure using AlphaFold 2 and RoseTTAFold 2, designed peptides to bind those predicted structures, and ended up solving the first structure of the pathogenic protein Rhombotarget A,” said co-lead author and graduate student Stephen Rettie.
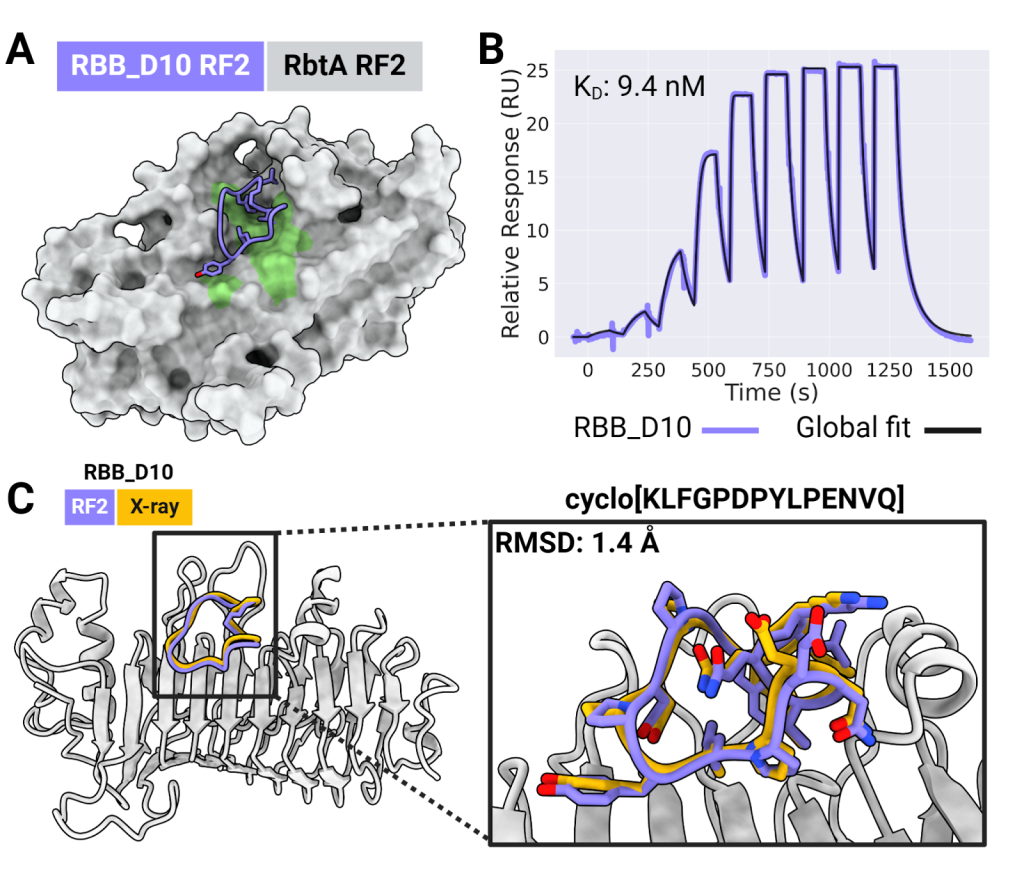
“This is quite a neat display of the robustness and generalization capacity these generative models gain during pretraining” said co-lead author David Juergens. “The implications for drug design are really exciting as macrocycles can be customized in many ways that normal peptides and proteins cannot.”
The development of RFpeptides marks another step in leveraging AI to solve complex challenges in biology. While initially tested as a drug design tool, RFpeptides could also be used to create diagnostic reagents and other custom peptides for research challenges beyond medicine.
This project included collaborators from MIT, Forschungszentrum Jülich, Heinrich Heine University Düsseldorf, University College Cork, Tufts University. All funders are listed in the manuscript, which is titled Accurate de novo design of high-affinity protein binding macrocycles using deep learning.