The de novo design of small proteins with beta-barrel topologies has been a challenge for computational design due to the complexity inherent in these folds. In a new study appearing in PNAS, a team led by Baker Lab research scientist David E. Kim describes the successful design and characterization of four different classes of small beta barrels using Rosetta energy-based methods and deep learning approaches.
The designed beta barrels exhibited high thermal stability and were observed to fold into their desired structures. This study provides insights into the determinants of folding and design of this important class of proteins and offers new routes to the design of high-affinity binding proteins by increasing the protein shape-space available for designing binders to protein targets of interest.
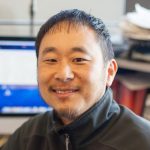
In the paper, the authors also discuss the relative strengths and weaknesses of traditional protein design using Rosetta and more recent deep-learning approaches, including hallucination. Their best results came from combining both methods: using hallucination for backbones and Rosetta for sequence design and structure relaxation. This combination led to well-folded proteins in 90 percent of cases compared with just 30 percent of cases when only Rosetta was used. However, recent work from the Baker Lab suggests that machine learning methods may soon take over the sequence design step as well.
Read the full report:
De novo design of small beta barrel proteins (Open Access)