Today we report in Nature [PDF] the computational design of highly efficient enzymes unlike any found in nature. Laboratory testing confirms that the new light-emitting enzymes, called luciferases, can recognize specific chemical substrates and catalyze the emission of photons very efficiently. This is an important step in the field of protein design as enzymes have many uses across biotechnology, medicine, environmental remediation, and manufacturing.
This project — which was powered by deep-learning software developed at the Institute for Protein Design — was led by two postdoctoral scholars in the Baker Lab, Andy Hsien-Wei Yeh and Christoffer Norn, and included collaborators in the Houk Lab at UCLA.
“Living organisms are remarkable chemists. Rather than relying on toxic compounds or extreme heat, they use enzymes to break down or build up whatever they need under gentle conditions. New enzymes could put renewable chemicals and biofuels within reach.”
— David Baker, Director of the Institute for Protein Design
To create new luciferases, the team first selected chemicals called luciferins that they wanted the proteins to act upon. They then used software to generate thousands of possible protein structures that might react with those chemicals. The best-performing enzymes emit enough light to be seen by the naked eye.
Hallucinating protein folds
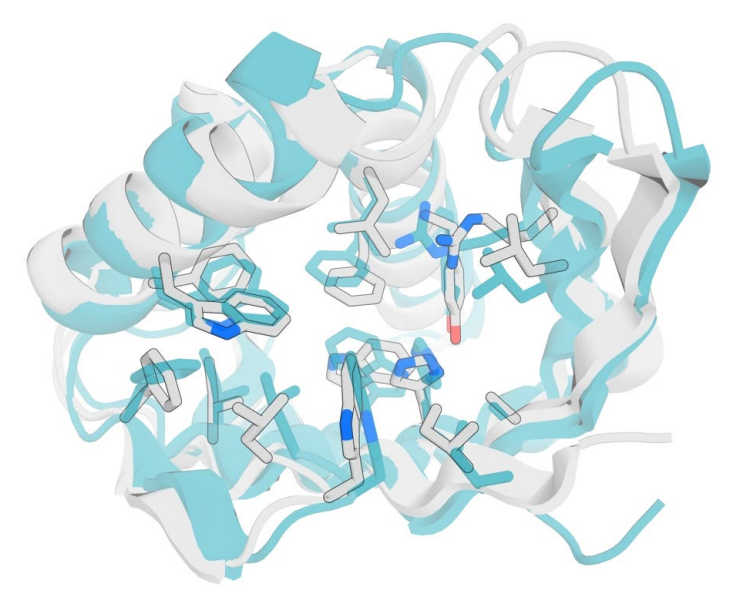
Rather than modifying existing proteins, the team devised a new deep-learning-based protein design strategy dubbed ‘family-wide hallucination.’ By integrating unconstrained de novo design and fixed backbone sequence-design approaches, this approach can generate an essentially unlimited number of never-before-seen proteins that have a desired fold.
Family-wide hallucination uses the de novo sequence and structure discovery capability of unconstrained protein hallucination for loop and variable regions, and structure-guided sequence optimization for core regions.
“Enzyme design has frustrated scientists for decades as it is one of the most challenging tasks in all of biochemistry. But with our new tools for generating fit-for-function scaffolds, I’m excited to see how much progress we can make in designing enzymes that have practical applications in medicine and biotechnology.”
– Christoffer Norn, co-lead author
To add active sites into the hallucinated protein folds, the team chose to precisely place a positively charged guanidinium group of an arginine residue to stabilize negative charges present on the reaction’s transition state. Additional active site residues were also designed.
Let there be light
When manufactured and tested in the laboratory, the researchers identified three active enzymes among their initial designs. They named the best-performing one LuxSit, a play on UW’s Latin motto lux sit, which roughly translates to ‘let light exist’.
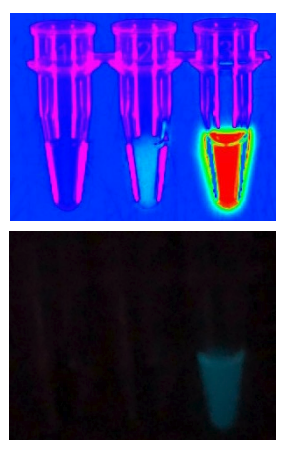
LuxSit has many properties that make it an attractive tool for biotechnological research. At just 117 residues, it is smaller than any known luciferase. Incubation of the enzyme with its synthetic luciferin substrate diphenylterazine (DTZ) resulted in blue luminescence at 480 nanometers, which is consistent with the substrate’s chemiluminescence spectrum. And the protein was found to remain partially folded under near-boiling conditions.
“We were able to design very efficient enzymes from scratch on the computer, as opposed to relying on enzymes found in nature. This breakthrough means that custom enzymes for almost any chemical reaction could, in principle, be designed.”
– Andy Hsien-Wei Yeh, co-lead author
Refinement of LuxSit led to dramatic improvements in performance. An optimized enzyme, dubbed LuxSit-i, generated enough light to be visible to the naked eye. It was found to be brighter than the natural luciferase enzyme found in the glowing sea pansy Renilla reniformis.
The power of deep learning
The team went on to design additional luciferases that recognize another synthetic luciferin substrate, 2-deoxycoelenterazine or h-CTZ.
Because the molecular shape of h-CTZ differs from DTZ, they again used family-wide hallucination to generate custom protein scaffolds. To create active sites, precise arrangements of histidine and arginine side chains were installed. These active site features were modeled after those observed to be most successful in the first round of luciferase design.
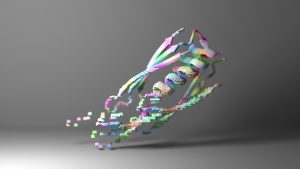
ProteinMPNN excels at creating new proteins
Rather than RosettaDesign, the team turned to the recently developed ProteinMPNN tool to come up with the remaining amino acid sequences of the new enzymes. ProteinMPNN is a sequence design tool powered by deep learning that runs in about one second, which is more than 200 times faster than the previous best software. Its results are superior to prior tools, and it requires no expert customization to run.
Out of the 46 designed h-CTZ catalysts tested in the lab, two were found to have measurable luciferase activity. This marks a hundred-fold increase in success rates — from 0.04% (3/7,648 for DTZ) to 4.35% (2/46 for h-CTZ) — for this second round of de novo enzyme design. This improvement is likely due to the knowledge gained during the first design round coupled with the increased performance of ProteinMPNN.
Until now, computational enzyme design had been limited by the number of available protein scaffolds and the extreme difficulty of placing enzyme active sites in them. The use of deep learning to produce large numbers of custom protein scaffolds, together with next-generation tools for protein sequence design, has set the stage for a new era in enzyme design.
Funding
This work was supported by the Howard Hughes Medical Institute, National Institutes of Health (K99EB031913), the United World Antiviral Research Network, National Institute of Allergy and Infectious Disease (1 U01 AI151698-01), Audacious Project at the Institute for Protein Design, Open Philanthropy Project Improving Protein Design Fund, Novo Nordisk Foundation (NNF18OC0030446), National Science Foundation (CHE-1764328, OCI-1053575), and Eric and Wendy Schmidt by recommendation of the Schmidt Futures program. The National Natural Science Foundation of China (22103060) provided partial computational resources.