Over the past two years, machine learning has revolutionized protein structure prediction. Now, three papers in Science describe a similar revolution in protein design. In the new papers, scientists in the Baker lab show that machine learning can be used to create proteins much more accurately and quickly than previously possible. This could lead to many new vaccines, treatments, tools for carbon capture, and sustainable biomaterials.
“Proteins are fundamental across biology, but we know that all the proteins found in every plant, animal, and microbe make up far less than one percent of what is possible. With these new software tools, we should be able to find solutions to long-standing challenges in medicine, energy, and technology,” said senior author David Baker.
To go beyond the proteins found in nature, our team broke down the challenge of protein design into three parts and used new software solutions, including ProteinMPNN, for each.
First, a new protein shape must be generated. In a paper published on July 21 in the journal Science, we showed that artificial intelligence can create new proteins that may be useful as vaccines, cancer treatments, or even tools for pulling carbon pollution out of the air. The team developed two strategies for designing new protein structures. The first, dubbed “hallucination,” is akin to DALL-E or other generative A.I. tools that produce output based on simple prompts. The second, dubbed “inpainting,” is analogous to the autocomplete feature found in modern search bars. “Most people can come up with new images of cats or write a paragraph from a prompt if asked, but with protein design, the human brain cannot do what computers now can,” said project scientist Jue Wang.
Second, to speed up the process, the team led by Justas Dauparas from the Baker lab devised a new algorithm for generating amino acid sequences. Described in the September 15 issue of Science, this software tool — called ProteinMPNN — runs in about one second, which is more than 200 times faster than the previous best software. Its results are superior to prior tools, and the software requires no expert customization to run. “Neural networks are easy to train if you have a ton of data, but with proteins, we don’t have as many examples as we would like. We had to go in and identify which features in these molecules are the most important. It was a bit of trial and error,” said project scientist Justas Dauparas.
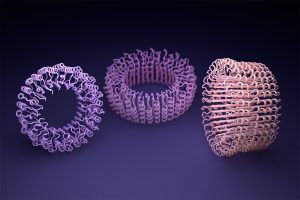
Third, we used AlphaFold, a tool developed by Alphabet’s DeepMind, to independently assess whether our designed amino acid sequences were likely to fold into the intended shapes. “Software for predicting protein structures is part of the solution but it cannot come up with anything new on its own,” explained Dauparas. “Even if you had a perfect tool for predicting how protein sequences fold, you would have to search through billions and billions of sequences to find any new useful proteins.”
“ProteinMPNN is to protein design what AlphaFold was to protein structure prediction,” said Baker.
In another paper appearing in Science, a team led by Basile Wicky, Lukas Milles, and Alexis Courbet from the Baker lab confirmed that ProteinMPNN together with the other new machine learning tools could reliably generate proteins that functioned in the laboratory. “It’s not enough to trust that the computer is designing proteins well — you have to actually study these molecules in the real world. We found that proteins made using ProteinMPNN were much more likely to fold up as intended, and we could create very complex protein assemblies using these methods” said project scientist Basile Wicky.
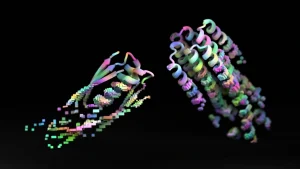
Among the new proteins made were nanoscale rings that the researchers believe could be used as parts for custom nanomachines. Electron microscopes were used to observe the rings, which have diameters roughly a billion times smaller than a poppy seed.
“This is the very beginning of machine learning in protein design. In the coming months, we will be working to improve these tools to create even more dynamic and functional proteins,” said Baker.
Funding
Compute resources for this work were donated by Microsoft and Amazon Web Services. Funding was provided by the Audacious Project at the Institute for Protein Design; Microsoft; Eric and Wendy Schmidt by recommendation of the Schmidt Futures; the DARPA Synergistic Discovery and Design project (HR001117S0003 contract FA8750-17-C-0219); the DARPA Harnessing Enzymatic Activity for Lifesaving Remedies project (HR001120S0052 contract HR0011-21-2-0012); the Washington Research Foundation; the Open Philanthropy Project Improving Protein Design Fund; Amgen; the Alfred P. Sloan Foundation Matter-to-Life Program Grant (G-2021-16899); the Donald and Jo Anne Petersen Endowment for Accelerating Advancements in Alzheimer’s Disease Research; the Human Frontier Science Program Cross Disciplinary Fellowship (LT000395/2020-C); the European Molecular Biology Organization (ALTF 139-2018), including an EMBO Non-Stipendiary Fellowship (ALTF 1047-2019) and an EMBO Long-term Fellowship (ALTF 191-2021); the “la Caixa” Foundation; the Howard Hughes Medical Institute, including a Hanna Gray fellowship (GT11817); the National Science Foundation (MCB 2032259, CHE-1629214, DBI 1937533, DGE-2140004); the National Institutes for Health (DP5OD026389); the National Institute of Allergy and Infectious Diseases (HHSN272201700059C); the National Institute on Aging (5U19AG065156); the National Institute of General Medical Sciences (P30 GM124169-01, P41 GM 103533-24); the National Cancer Institute (R01CA240339); the Swiss National Science Foundation; the Swiss National Center of Competence for Molecular Systems Engineering; the Swiss National Center of Competence in Chemical Biology; and the European Research Council (716058).